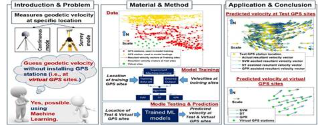
Machine learning techniques used by scientists for modelling crustal deformations on the Tibetan Plateau have helped predict the velocity vectors of such movements and enhance characterisation of plate movements. Typically, a dense network of continuously operating reference stations (CORS) is created to continuously monitor crustal deformation.
Campaign-mode GPS surveys are often used to densify existing CORS networks. Installing stations at the desired location can be very challenging due to logistics problems and regional geographic importance. Moreover, the process is expensive, and studies on crustal activity are often hampered by data scarcity due to logistical restrictions. Machine learning techniques can come as an aid in predicting GPS site velocities for crustal deformation research in such situations.
To obtain velocity vectors at the desired locations, scientists from the Wadia Institute of Himalayan Geology, an autonomous institute under the Department of Science and Technology (DST), Government of India, adopted machine learning techniques such as Support Vector Machines, Decision Trees and Gaussian Process Regression to accurately model the crustal activity.
The scientists analyzed data from 1,271 permanent continuous and expedition-mode GPS stations located in and around the Tibetan Plateau. They used data from 892 stations for model training and 379 stations for testing.